最近刚结束一场McKinsey的数据工程岗位远程面试,干货太多,不写出来我怕自己忘了 😂 这篇文章就来带大家一起走一遍完整面试流程,尤其适合正在准备McKinsey技术岗的小伙伴!
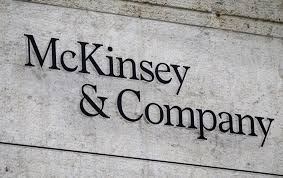
🧑💻 面试背景介绍
- 公司:McKinsey & Company
- 岗位方向:Scientific AI / Data Engineering
- 面试形式:远程 + Hackerrank协作平台实时coding
- 面试时长:约50分钟
- 语言:全程英文,考察技术理解和现场表达
面试官是McKinsey内部Scientific AI组的工程师,非常友好,开场就直接介绍流程:“我们有三道题,Python / SQL / Pandas任选顺序,预计每题15分钟左右。”流程清晰,时间控制也很紧凑。
💡 面试题目一:Data Cleaning 谁是最后出手的工程师?(逻辑题)
🧠 原题如下:
In a text data cleaning pipeline, two engineers alternate removal of substrings:
- Alex removes substrings with an odd number of vowels.
- Chris removes substrings with an even number of vowels.
- Alex goes first, and they alternate turns optimally until no valid substrings remain.
Write a function
optimizeDataCleaning(datasets: List[str]) -> List[str]
to determine who removes the last substring.
✅ 解题核心:
这道题表面是“字符串操作”,本质是“博弈模拟”!
🌟 关键点:
- 每个字符串中,统计子串(或整体)中元音数量
- 根据“奇偶”轮流删除,直到无法删除
- 谁最后一次成功删除谁赢
💬 面试实录精华:
我现场先和面试官确认了规则,随后快速提出策略:只要能统计出总共有多少“可删除子串”,轮流模拟,谁最后动手谁赢。我写了一个 count_vowels
函数用来统计元音,然后写了完整轮转逻辑。
面试官看我能把逻辑说清楚,没太纠结具体实现的小bug,非常友好地指出:“你快写完了,继续就行”。
🧾 面试题目二:SQL 部门员工数量统计
🧠 原题如下(英文):
Given two tables, Employee and Department, generate a summary of how many employees are in each department. Each department should be listed, even if they have 0 employees.
Sort results by:
- Employee count (descending)
- Department name (alphabetical)
✅ 解题思路:
典型的 LEFT JOIN + GROUP BY
题型:
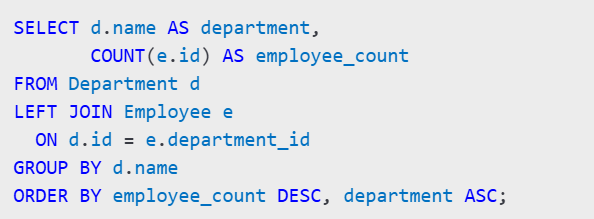
🐼 面试题目三:Pandas 多列数据清洗与特征统计
🧠 原题如下:
Given a DataFrame containing restaurant data:
- Drop restaurants that offer fewer than 3 cuisines.
- Clean the
location_code
column so that it only contains alphanumeric characters.- For each location, count the number of restaurants offering:
- Italian
- Chinese
- Indian
- Mexican
Each cuisine should be in a separate column in the output DataFrame.
✅ 解题策略:
str.split()
→ 统计菜品数目- 用正则 or
apply
+join
去除非法字符 apply(lambda x: cuisine in x)
生成多个one-hot列groupby(location_code).sum()
聚合结果!
💬 面试实录精华:
这个题写得比较赶,时间只剩10分钟。但我还是一步步拆解逻辑,Pandas链式操作一把梭。
❓面试官互动环节
- 我提问:“Scientific AI在McKinsey未来的战略角色是什么?”
- 面试官解释得非常清楚:这个组主要做生命科学相关AI建模,和R&D部门客户合作,正处于快速扩展阶段。
- 他强调:“虽然叫Scientific AI,但岗位本质是非常工程导向的,写代码、做建模、优化pipeline是核心工作。”
✍️ 总结:McKinsey面试感受 & 建议
- ✅ 技术面务实、专业、友好:没有压迫感,所有题都能通过交流解决,面试官也会主动引导你思考。
- 🧠 题目难度中等偏上:需要具备扎实的Python/Pandas/SQL基本功,和一定的实战拆题能力。
- 🧍 英文表达很重要:需要边写边说,逻辑表达+技术词汇都要过得去。
- ⏳ 时间紧凑,要合理分配:题目是“足够做完的量”,但不能浪费时间debug细节。
如果你也正在准备McKinsey或者其他咨询公司的数据工程面试,不妨模拟这类真实Coding Round场景,不仅要会写,还要会说,特别是解释你的思路比答案本身更关键!
🔥祝大家都能稳稳拿下心仪的offer!
经过csoahelp的面试辅助,候选人获取了良好的面试表现。如果您需要面试辅助或面试代面服务,帮助您进入梦想中的大厂,请随时联系我。
If you need more interview support or interview proxy practice, feel free to contact us. We offer comprehensive interview support services to help you successfully land a job at your dream company.
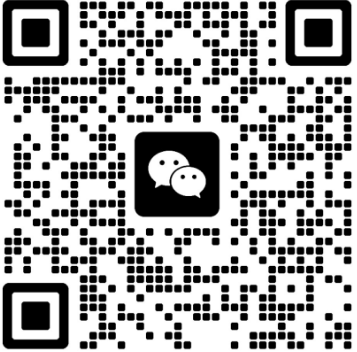